Imagine you have a robot friend that knows a ton of things but can sometimes get stumped on questions it hasn’t encountered before. When that happens, instead of just guessing or giving a vague answer, this friend can quickly visit a library to fetch the correct information. This clever combination of “guessing” from what it knows and “checking” with real-time information retrieval is what RAG (Retrieval-Augmented Generation) does!
What is RAG?
RAG, or Retrieval-Augmented Generation, is a super-smart AI method that enhances how AI models answer questions. Traditional AI models were limited by what they had already been trained on, meaning they could only answer based on their prior knowledge. RAG changes this by letting AI models “visit” an external source of information in real-time when they don’t have an answer at hand. This means they can keep up with current events, industry-specific data, or any rapidly changing field, giving answers that are both accurate and timely.
In simpler terms, RAG makes an AI act like your best friend who doesn’t just know everything but also knows where to look if it’s unsure. It’s like a big brain combined with a library card!
A Little Bit of History
People have been trying to build talking robots for a long time. Early AI models could only answer questions based on what they’d been trained on – imagine a robot that has read a bunch of books but can’t look up anything new. As more complex questions started being asked, people realized these AI models needed a way to look up new information.
RAG was born from this need to make AI smarter. Instead of just reading books and guessing, it was like giving AI a way to pop into a library for extra info if it wasn’t sure about something. Now, thanks to RAG, AI models can retrieve up-to-the-minute information and generate responses that reflect the latest knowledge, not just what they’ve memorized.
How Does RAG Work?
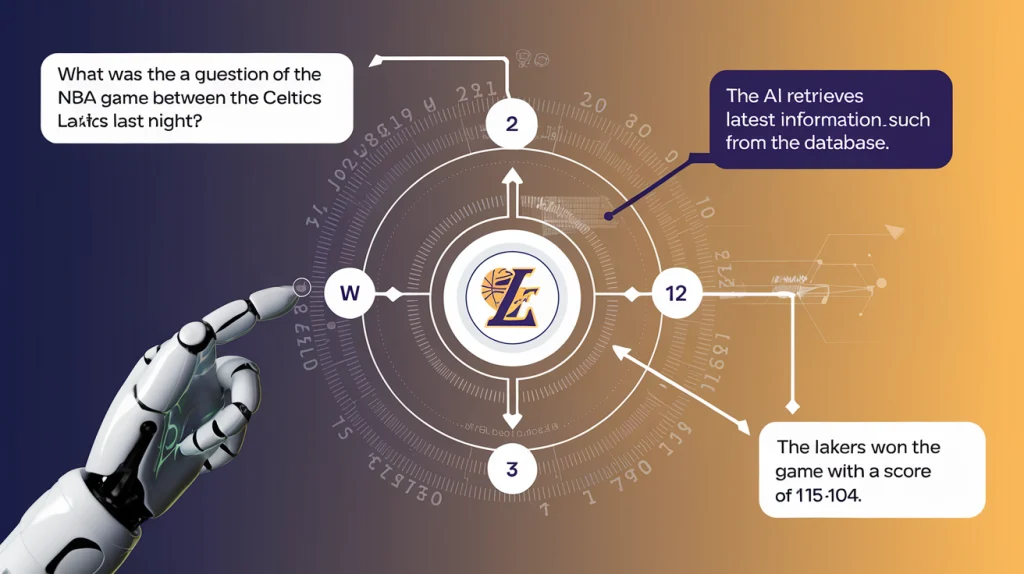
Think of RAG as a two-part system:
- Guessing from Memory: When you ask a question, the RAG system first tries to answer based on what it already “knows” from its training data.
- Checking the Library: If it doesn’t find a satisfying answer, RAG activates a special tool to “look up” more information. This external library, or database, is filled with the latest and most relevant information. The RAG system retrieves this info and combines it with its own knowledge to give you a solid, accurate answer.
So, if you ask a RAG model, “What’s the weather like today?” it can quickly check a database for current weather data, rather than simply telling you general weather patterns it has learned from past data.
Example of RAG in Real Life
Imagine you’re a sports fan and ask your robot friend, “Who won yesterday’s football game?” Normally, an AI trained a month ago would struggle with this question because it doesn’t know yesterday’s events. But with RAG, the robot can check a live sports database, retrieve the latest score, and respond with the correct answer, almost as if it attended the game itself!
This example is why RAG is becoming crucial in applications like news sites, customer service, and search engines. Whenever up-to-date, accurate information is needed, RAG provides it instantly.
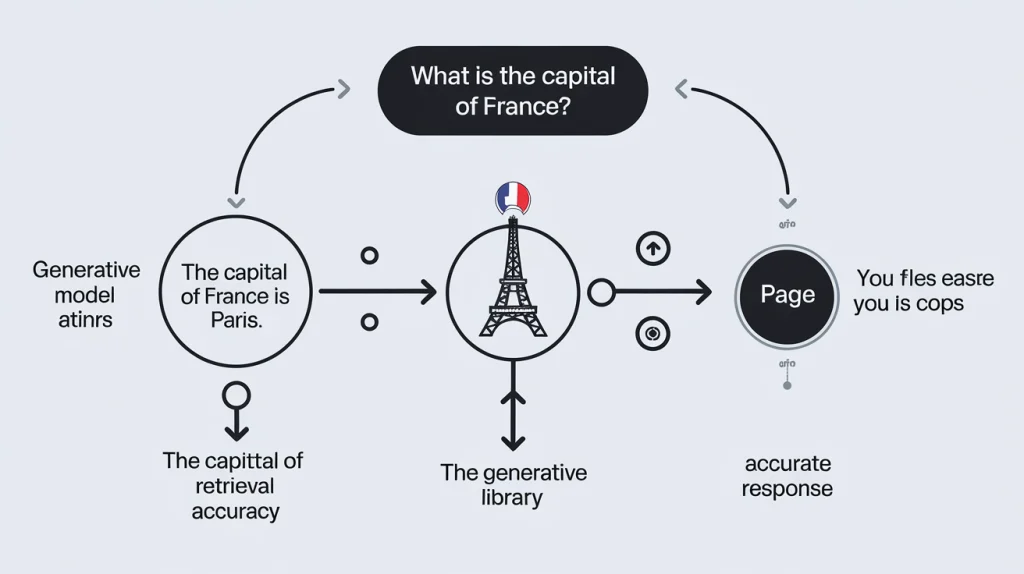
Why Do Companies Use RAG?
Many industries and companies use RAG for its ability to quickly find and deliver precise answers. Here’s a list of a few key areas where RAG is already making an impact:
- News Outlets: News sites use RAG to keep up with breaking stories, so readers get the latest and most accurate information.
- Customer Support: Imagine a customer service bot that can check a company’s policies in real-time, retrieving the exact details a customer needs to know.
- Healthcare and Research: Medical databases change rapidly as new research is published. RAG allows medical AI systems to retrieve up-to-date research findings to answer questions accurately.
- Education: Students and researchers can use RAG-based tools to find the latest articles, research papers, and even experiment results without having to search manually.
In short, RAG has become the go-to choice for any field that requires reliable, updated, and context-sensitive answers.
The Benefits of Using RAG
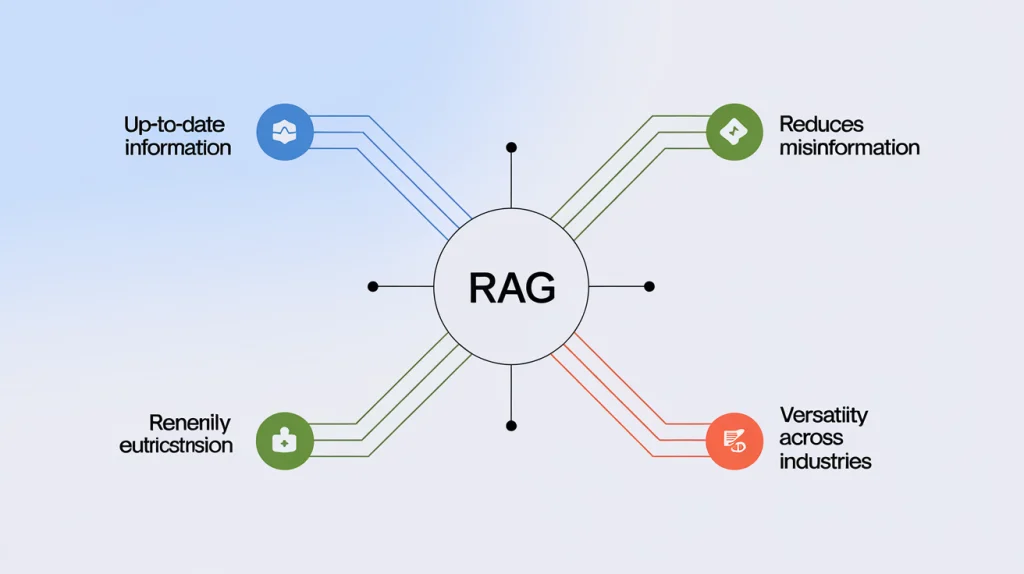
1. Up-to-Date Information
Because RAG has access to real-time information, it can respond to questions with the most recent data. This is especially valuable in rapidly changing fields like medicine, technology, and news.
2. Reduces Misinformation
Traditional AI could sometimes mislead by producing outdated information. RAG reduces this risk by checking current data sources, making it a powerful tool against misinformation.
3. Versatile Across Industries
Since RAG can pull information from specific databases, it’s useful for various industries, including finance, education, legal, and entertainment.
How Does RAG Fit into the Bigger Picture of AI?
RAG sits at the forefront of what’s called generative AI. Traditional generative AI models create responses or content based on a vast amount of training data, but they don’t keep updating with new information. RAG, on the other hand, takes this one step further by combining generative abilities with a retrieval system, making it more adaptable and flexible.
In the world of AI architecture, this unique setup makes RAG an “AI agent” that not only generates responses but also validates them in real-time with updated data.
Fun Fact: RAG is Always Learning
Every time RAG retrieves information from its library, it learns a little more about where to find the right answers. This isn’t exactly the same as “learning” in the way humans do, but over time, RAG systems can improve their efficiency, pulling the best answers faster and becoming more accurate. So, in a way, RAG isn’t just giving answers—it’s also growing and adapting over time.
FAQs: What Else Should You Know to Be Knowledgeable About RAG?
1. What does RAG stand for in AI?
- RAG stands for Retrieval-Augmented Generation, a technique combining a model’s own knowledge with up-to-date data retrieval.
2. What makes RAG different from other AI models?
- Unlike traditional models that rely only on pre-trained data, RAG uses a retrieval system to pull in new information, enhancing both accuracy and relevance.
3. What is RAG AI architecture?
- The architecture includes both a retrieval mechanism (to search for answers) and a generative model (to synthesize responses), making it highly versatile.
4. Why is RAG important for AI?
- It provides timely, accurate answers that keep up with changing information, which is critical in areas like finance, news, and customer service.
Conclusion: Why RAG Matters
RAG’s ability to mix real-time retrieval with generation makes it one of the most exciting advancements in AI today. Imagine having a personal assistant that isn’t just knowledgeable but also aware of the latest developments in any field. That’s RAG—a new standard in AI, offering precision and intelligence on demand.
Whether you’re in healthcare, education, or just a curious tech enthusiast, RAG can open up possibilities in AI that traditional systems couldn’t. And as it continues to develop, RAG is set to become a cornerstone of AI, making interactions with machines feel more intuitive, accurate, and dynamic than ever.
In the growing landscape of generative AI, knowing about RAG gives you a glimpse into the future of smarter, more reliable AI systems.